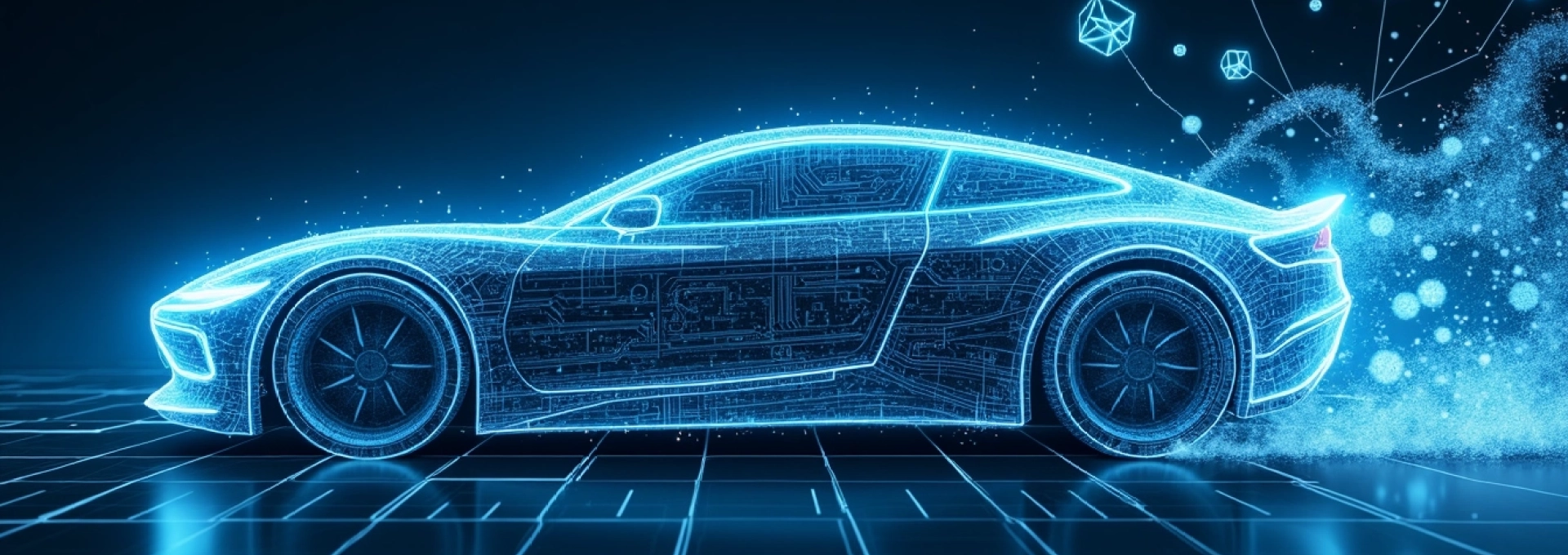
Artificial intelligence (AI) is revolutionizing the automotive industry, ushering in a new era of vehicle design and engineering. From optimizing aerodynamics to discovering advanced materials, AI is reshaping how cars are conceptualized, developed, and manufactured. This transformative technology is enabling designers and engineers to push the boundaries of innovation, creating vehicles that are not only more efficient and safer but also more aesthetically pleasing and user-centric.
The integration of AI in vehicle design is driving significant changes across the entire automotive value chain. By leveraging machine learning algorithms, generative design techniques, and advanced simulations, manufacturers are able to iterate designs faster, reduce costs, and bring cutting-edge vehicles to market in record time. This AI-driven approach is not just about automating existing processes; it's about unlocking new possibilities and solving complex challenges that were previously insurmountable.
Machine learning algorithms in vehicle design optimization
Machine learning algorithms are at the forefront of vehicle design optimization, offering unprecedented capabilities in analyzing vast amounts of data to identify patterns and make predictions. These algorithms can process information from multiple sources, including historical design data, performance metrics, and real-world driving conditions, to generate optimized design solutions.
One of the key advantages of using machine learning in vehicle design is its ability to rapidly explore a wide range of design possibilities. Traditional design methods often rely on iterative processes that can be time-consuming and limited by human cognitive capabilities. In contrast, machine learning algorithms can evaluate thousands of design variations simultaneously, considering complex interactions between different components and systems.
For example, machine learning can be applied to optimize the shape of vehicle bodies for improved aerodynamics. By analyzing data from wind tunnel tests and computational fluid dynamics (CFD) simulations, AI algorithms can suggest subtle modifications to the vehicle's contours that can significantly reduce drag and improve fuel efficiency. This level of optimization would be extremely challenging and time-consuming to achieve through conventional methods alone.
Moreover, machine learning algorithms can continuously learn and improve from new data, making them invaluable tools for ongoing design refinement. As more data is collected from prototypes, test drives, and even vehicles in use, these algorithms can provide increasingly accurate and sophisticated design recommendations.
Ai-driven generative design for automotive components
Generative design, powered by AI, is revolutionizing the way automotive components are created. This innovative approach uses algorithms to explore all possible permutations of a solution, generating design options that humans might never consider. By inputting design goals and constraints, such as weight limits, strength requirements, and manufacturing methods, AI can produce multiple design iterations that meet or exceed specified criteria.
The impact of generative design on automotive engineering is profound. It allows for the creation of parts that are lighter , stronger , and often more aesthetically pleasing than their conventionally designed counterparts. This is particularly crucial in the era of electric vehicles, where weight reduction is a key factor in extending range and improving performance.
Topology optimization using Autodesk fusion 360
Autodesk Fusion 360 is a powerful tool that integrates AI-driven topology optimization into the design process. Topology optimization is a mathematical method that optimizes material layout within a given design space, for a given set of loads and boundary conditions. When combined with AI, this process becomes even more powerful.
Using Fusion 360's generative design capabilities, automotive engineers can input specific requirements for a part, such as mounting points, load-bearing areas, and material constraints. The AI then generates multiple design options, each optimized for factors like weight reduction, stress distribution, and manufacturability. This approach has led to the creation of components that are up to 40% lighter than traditional designs while maintaining or even improving structural integrity.
Siemens NX and AI-enhanced CAD modeling
Siemens NX, a leading computer-aided design (CAD) software, has incorporated AI to enhance its modeling capabilities. The integration of AI in Siemens NX allows for more intuitive and efficient design processes. For instance, the software can now predict designer intent, suggesting next steps or autocompletion of common design features based on historical data and learned patterns.
This AI-enhanced CAD modeling significantly reduces the time spent on repetitive tasks, allowing designers to focus on more creative aspects of vehicle design. It also helps in maintaining consistency across complex assemblies, as the AI can automatically apply design rules and standards across multiple components.
Nvidia's AI-assisted rendering for concept visualization
NVIDIA's AI technologies are transforming the way automotive designers visualize and refine their concepts. Using deep learning algorithms, NVIDIA's rendering tools can produce photorealistic images of vehicle designs in real-time. This capability allows designers to see their ideas come to life instantly, with accurate lighting, materials, and environmental reflections.
The impact of this technology on the design process is significant. Designers can now iterate much faster, experimenting with different colors, materials, and finishes without the need for time-consuming physical prototypes. This not only speeds up the design process but also allows for more creative exploration and refinement of concepts before committing to physical production.
Computer vision in aerodynamics and aesthetics
Computer vision, a branch of AI that enables machines to interpret and understand visual information, is playing an increasingly important role in vehicle design, particularly in the realms of aerodynamics and aesthetics. By analyzing images and video data, computer vision algorithms can provide insights into how air flows around a vehicle and how different design elements contribute to its overall visual appeal.
In aerodynamics, computer vision is used in conjunction with wind tunnel testing and CFD simulations to provide a more comprehensive understanding of a vehicle's performance. High-speed cameras capture the movement of air around the vehicle, and AI algorithms analyze this visual data to identify areas of turbulence or inefficient airflow. This information can then be used to refine the vehicle's design for improved aerodynamic efficiency.
CFD simulations with OpenFOAM and TensorFlow
The combination of OpenFOAM, an open-source CFD software, and TensorFlow, Google's machine learning platform, is revolutionizing aerodynamic simulations in vehicle design. This integration allows for more accurate and efficient simulations of complex airflow patterns around vehicles.
By training neural networks on vast datasets of CFD simulations and real-world testing data, AI can predict airflow patterns with remarkable accuracy. This not only speeds up the simulation process but also allows for the exploration of novel design concepts that might be overlooked in traditional CFD approaches. The result is vehicles with optimized aerodynamics that contribute to improved fuel efficiency and performance.
Real-time shape optimization using ANSYS Discovery Live
ANSYS Discovery Live is a groundbreaking simulation tool that leverages AI to provide real-time feedback on design changes. This technology allows designers to see the immediate impact of their modifications on factors like aerodynamics, structural integrity, and thermal management.
The real-time nature of Discovery Live enables a more iterative and exploratory design process. Designers can quickly test multiple variations of a shape or component, instantly visualizing how these changes affect the vehicle's performance. This rapid feedback loop accelerates the design optimization process and leads to more innovative and efficient vehicle designs.
Bmw's implementation of AI in design language evolution
BMW has been at the forefront of integrating AI into its design processes, particularly in the evolution of its design language. The company uses AI algorithms to analyze historical design data, consumer preferences, and market trends to inform its future design directions.
One notable application is in the refinement of BMW's signature kidney grille. AI algorithms analyze the visual impact of different grille designs, considering factors such as brand recognition, aerodynamic performance, and aesthetic appeal. This data-driven approach helps BMW designers create grilles that are not only visually striking but also functionally optimized.
Natural language processing for user-centric design
Natural Language Processing (NLP), a branch of AI that focuses on the interaction between computers and human language, is playing an increasingly important role in creating user-centric vehicle designs. By analyzing vast amounts of textual data from customer feedback, social media, and industry publications, NLP algorithms can provide designers with valuable insights into user preferences, pain points, and emerging trends.
This application of AI in vehicle design goes beyond mere aesthetics. It helps manufacturers understand how users interact with their vehicles, what features they value most, and what improvements they desire. For instance, NLP can analyze customer reviews to identify common complaints about interior layout or control placement, informing ergonomic improvements in future models.
Moreover, NLP is enabling more intuitive voice-activated controls in vehicles. By understanding natural language commands and context, these systems can provide a more seamless and user-friendly interface between driver and vehicle. This not only enhances the user experience but also contributes to safety by reducing driver distraction.
The integration of NLP in vehicle design is transforming cars into more responsive and personalized machines, attuned to the needs and preferences of their users.
AI in material science and vehicle engineering
Artificial intelligence is making significant strides in materials science, a field crucial to vehicle engineering. AI algorithms are being used to discover and develop new materials with properties tailored for specific automotive applications. This includes materials that are lighter, stronger, more durable, or have better thermal or electrical properties than conventional options.
The impact of AI in materials science extends to every aspect of vehicle design, from body panels and structural components to battery technology in electric vehicles. By accelerating the discovery and testing of new materials, AI is enabling manufacturers to create vehicles that are not only more efficient and environmentally friendly but also safer and more resilient.
IBM Watson's role in advanced materials discovery
IBM Watson, a powerful AI system, has been applied to the field of materials science with remarkable results. Watson's ability to process and analyze vast amounts of scientific literature and experimental data allows it to identify patterns and relationships that human researchers might overlook.
In the context of vehicle engineering, Watson has been used to explore new alloys and composite materials that could revolutionize automotive construction. For example, it has helped in the development of lightweight yet strong materials that can improve fuel efficiency without compromising safety. Watson's insights have also contributed to the advancement of battery technology, a critical component in the evolution of electric vehicles.
Machine learning for predictive maintenance and structural analysis
Machine learning algorithms are transforming how vehicles are maintained and how their structural integrity is analyzed over time. By processing data from sensors embedded throughout a vehicle, these algorithms can predict when components are likely to fail, allowing for proactive maintenance that extends the life of the vehicle and improves safety.
In structural analysis, machine learning models can simulate the long-term effects of stress and fatigue on vehicle components. This allows engineers to design parts that are more durable and reliable, reducing the likelihood of failures and improving overall vehicle longevity. The predictive capabilities
of these AI models are particularly valuable in the development of autonomous vehicles, where reliability is paramount.
AI-optimized composite materials in Tesla's Cybertruck design
Tesla's Cybertruck showcases the potential of AI-optimized composite materials in vehicle design. The truck's exoskeleton is made from an ultra-hard 30X cold-rolled stainless steel, a material choice that was likely informed by AI analysis of various alloys and their properties.
AI algorithms were likely used to optimize the composition and structure of this material, balancing factors such as strength, weight, and manufacturability. The result is a vehicle that breaks convention in both its appearance and its engineering, demonstrating how AI can lead to innovative solutions in automotive design.
Autonomous systems and their impact on vehicle form factor
The advent of autonomous driving technology is fundamentally changing the way vehicles are designed. As the need for human operation diminishes, the entire concept of a vehicle's form factor is being reimagined. AI is at the heart of this transformation, not only in the development of autonomous systems themselves but also in rethinking vehicle layouts and interiors.
For instance, AI algorithms are being used to optimize the placement of sensors and cameras required for autonomous operation, integrating them seamlessly into the vehicle's design. This challenge requires balancing functional requirements with aesthetic considerations, a task well-suited to AI's ability to process multiple variables simultaneously.
Moreover, the shift towards autonomous vehicles is prompting a rethinking of interior spaces. With the driver no longer needing to focus on the road, interiors can be designed for comfort, productivity, or entertainment. AI is being employed to analyze user behavior and preferences to create adaptable interior spaces that can transform based on the needs of the occupants.
The integration of autonomous systems is not just changing how cars drive, but fundamentally altering what a car can be.
As AI continues to advance, its role in vehicle design will only grow more significant. From optimizing aerodynamics and discovering new materials to reimagining the very concept of what a vehicle can be, AI is driving the automotive industry towards a future of unprecedented innovation and efficiency.